Self Driving Car Ethics: Beyond A Glorified Trolly Problem
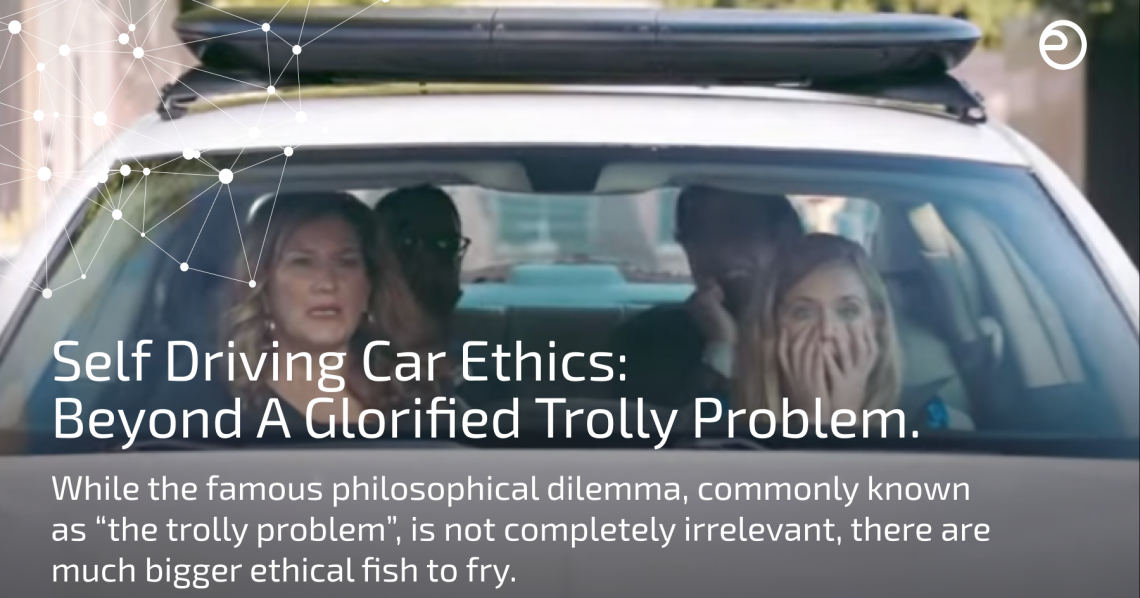
The self-driving cars ability to accurately decide on which lives to save and which to sacrifice very much depends on its ability to detect each and every one of those lives to begin with.
Is there a risk of self-driving cars identifying some pedestrians but not others? The short answer is yes, there is. While the famous philosophical dilemma, commonly known as “the trolly problem”, is not completely irrelevant, there are much bigger ethical fish to fry.
Picture this. December 2021 the pilot episode of the NBC sitcom “American Auto” airs. In the episode, the Detroit-based fictional company “Payne Motors” is rolling out its new self-driving car (SDC). While the team gives the newly hired CEO a ride in the SDC on the on-site training course, the car, which successfully stopped for the “test pedestrians”, runs over one of Payne Motors African-American employees. Later in the episode, the team discusses how the machine learning (ML) model “sometimes confuses dark items with shadows” and how all the “test pedestrians” the SDC was trained on were, well….white.
The SDCs ability to accurately decide on which lives to save and which to sacrifice very much depends on its ability to detect each and every one of those lives to begin with.
Is there a risk of SDCs identifying some pedestrians but not others? The short answer is yes, there is.
Photo credit: Sudip Das – ClueNet – CityPersons
A recent study that examined the accuracy of 8 detection algorithms on 4 datasets found that on average the models have a 7-10% higher miss rate on dark-skinned pedestrians and 19-26% higher miss rate on children. Another study by the University of Oxford also found that children and women had higher miss rates than adults and men. A study by Stony Brooke University also found evidence of worse performance when predicting the pedestrian trajectories of children and the elderly compared to adults. The potential for bias is real, and the potential for real violation of human well-being is real.
What can be done about this risk? AI is reliant on Machine Learning (ML) models and training data. There are multiple points within the ML lifecycle where bias can be introduced or created, typically starting with the data labeling phase. Data labeling is critical to AI success, it ensures training data accuracy by making objects recognizable and understandable for the model. The labeling of the training data is what the model will use as a basis for identifying and categorizing objects. If there is bias in the data or labeling practices, the AI will learn to reflect, and often magnify, that bias. Lack of sufficient representation in training data and labeling practices is no foreign concept to AI ethical issues and can lead to significant unfair outcomes.
FAIRNESS THROUGH BLINDNESS AND REDUNDANT ENCODINGS
While some argue that including sensitive attributes may harm the fairness of the model’s predictions, it has been proven to do the opposite. As mentioned in The Alignment Problem, removing a sensitive label, such as race or gender, can harm the fairness of the model. Fairness through blindness does not work.
The book states that it is one of the most robust and established findings of AI Ethics research. Removing the sensitive attribute not only makes it impossible to measure for bias, but to mitigate it as well. Removing sensitive attributes (race, gender) does nothing to contribute to fairness and often makes it impossible to evaluate bias and mitigate it. For example, when Amazon was developing an AI tool to review resumes, they quickly noticed that words like “women’s” were being penalized. The model was edited, and they noticed it started to assign negative scores to all women collages. The model was edited again, and they noticed it started assigning positive scores to vocabulary and word choices more prevalent in men’s resumes.
This concept is known as “redundant encodings”, protected attributes are typically redundantly encoded across other variables. It is also important to keep protected attributes to account for special circumstances. A model that is trained to assign a penalty to candidates who did not have a job in the prior year will not be able to take into account variables like pregnancy or maternity leave if a variable with such a high correlation to pregnancy and childbirth (gender) is removed or if the model is “gender-blind”.
A quick look at the annotation practices used for Autonomous Vehicle training showed that there is evidence of potential disparities caused by insufficient labeling practices.
NUSCENES
The popular SDC training data set provided by Nuscenes features labels for:
- “human.pedestrian.adult”
- “human.pedestrian.child”
- “human.pedestrian.construction_worker”
- “human.pedestrian.personal_mobility”
- “human.pedestrian.police_officer”
- “human.pedestrian.stroller”
- “human.pedestrian.wheelchair”
But nothing gender or race related.
Another sobering component of this project is where the data is collected from. The website clearly states that the US dataset is collected from the Boston Seaport. This is a location where the population is reported to be 2% black, 82% white, with a median household income of $167,000. Hardly representative of the US population, and definitely not diverse enough to bypass fairness testing.
WAYMO
Waymo on the other hand, simply labels all pedestrian instances as “TYPE_PEDESTRIAN” with no further sub-categorization (Waymo Labeling – GitHub). Which again raises the question, how are you testing your dataset for racial or gender bias if you have no labels for either?
Where does Waymo gather data from? Perhaps the locations or areas chosen are highly diverse. They are not. Waymo gathers its data from four cities according to their open dataset documentation. Table 1 below shows each city with its population distribution according to the US census.
City | Racial Demographics according to census.gov | Median Household Income | |
64.4% White | 7.3% Black | $64,927 | |
71.2% White | 1.7% Black | $121,998 | |
48% White | 2.4% Black | $158,104 | |
43% White | 5.2% Black | $126,187 |
Table 1 – Waymo data collection cities US census data
For reference, the median household income in the US is $69,021. And the median household income for the city of Detroit, which is known as “the motor city”, is $34,762 (12.9% white, 77.9% black).
The interesting thing is that Waymo has a published statement regarding the ”wide” variety of its dataset.
The Waymo Open Dataset covers a wide variety of environments from dense urban centers to suburban landscapes, as well as data collected during day and night, at dawn and dusk, in sunshine and rain. – Waymo
While diversifying your training data with urban and suburban landscapes, day and night time, and rain and sunshine is important, other components are equally as critical. Where are the rural landscapes? Where is the diversity in pedestrian skin colors and ages? Where is the diversity regarding communities with lower income levels? Is there any training data representing weather such as snow?
TESLA
If you are relying on data gathered from existing drivers of your vehicles (for example all Tesla owners) you are still running into the same type of problem where the locations the vehicles travel through will not be particularly diverse since this is a particularly pricey technology.
55% of Tesla Model 3 owners live in the wealthiest 10% of ZIP codes in the United States. The ethnicity of Tesla owners skews toward Caucasians, at 87%. Owners who identify with Hispanic ethnicity make up 8% of Tesla owners, leaving 5% to other ethnicities.(Source)
While the reality may not be quite as dramatic as a scene in a satirical television series, the potential for unfair outcomes perpetuated by biased datasets and labeling practices in SDCs is very much real. A transparency-based solution towards minimizing bias through AI model and training data evaluation can help mitigate the risk associated with these challenges.
THE OPEN ETHICS DATA PASSPORT
Photo credit: Nikita Lukianets – Open Ethics Data Passport
The Open Ethics Data Passport provides insight into the origins of the models, the dataset acquisition methods, the data type (synthetic, human-generated), the labeling practices, and labelers’ profiles.
It is an iterative tool built to provide in-depth transparency and awareness and can help tackle issues of bias that lead to unfair outcomes in any AI use case, including Self-Driving Cars. It can be used on any AI product and its components throughout the product stages of development, including in the early stage of system design.
The OEDP is a part of the nutrition label for AI. It is responsible for a deeper level of transparency, where the ingredient, in this case the AI product, is broken down through disclosure of sub-ingredients (AI components of the product). This provides a powerful level of insight and transparency that aids in identifying systematic bias in trained AI models.
For more information on the Open Ethics Data Passport, you can visit the project or the Github page. And if you would like to become a contributor or a member of Open Ethics Initiative, you can apply here! 🙂
Data labeling practices can heavily influence the level of model bias, and as a result cause potentially unfair outcomes. Mitigating these potential biases is necessary for the prevention of inevitable feedback loops within AI models, and in systems with a direct impact on human life, such as SDC pedestrian detection, it is of critical importance.
Featured Photo Credits: American Auto – Pilot (NBC) – Watch Clip